In the past decade, finance and investment firms have increasingly deployed ever-more sophisticated AI technology to transform and automate their operations across multiple areas, from the back-end to the front.
During this next decade, AI’s advance will only accelerate, powered by new, emerging technologies such as Natural Language Processing, which promise to revolutionize financial institutions’ use of data, unlocking new actionable insights that enable them to gain a sharper, more competitive edge.
To discuss this development, and specifically the rise in use of NLP technology and alternative data, GlobalCapital and S&P Global convened a panel of experts to tackle the topic and explain where and how NLP is being deployed during a live webinar.
The full recorded webinar session can be viewed below. Alternatively, the replay can be viewed on the BrightTalk webinar platform (click on the hyperlink to take you to the webinar page).
The experts were:
Gabriel Andraos, Co-Head of Voya Machine Intelligence (VMI), Research & Development, Voya Investment Management
Pietro Casella, Managing Director at EQT Group and Chief Architect of EQT Digital and Motherbrain Labs
Rob Oddy, Enterprise Architecture Executive for Global Technology, Bank of America
Michael Hoffmann, Head of Business Development, Kensho Technologies, S&P Global's AI innovation hub
Chirag Patel, a senior member of the EMEA Desktop Business Development team, S&P Global Market Intelligence
The high-level discussion covered a lot of ground. Key points have been distilled below.
Where is the financial industry on AI, and what has changed since last year?
EQT's Casella said there are probably four key factors influencing AI’s rise in financial services today: public and board-level awareness of AI and its uses has risen sharply, which drives demand; AI solutions are better packaged and performance is demonstrably more useful and valuable; AI models as a service have become commoditized and more widely available; as a result of all this, there has been an explosion in innovation and rapid expansion in the number and variety of use cases.
Voya's Andraos added that this represents a Promethean moment, which has both positive and negative implications for the future. Focusing on the positives, the advance of NLP will help us “take care of some of the grunt work”, with new programming paradigms completely changing the way people interact with computers using natural language, which is going to increase productivity and potentially job happiness.
“I remember being a junior investment banker back in the 1990s. I know that ChatGPT style technologies can do a lot of the work I used to do back then. That's not a bad thing,” he said
How can financial institutions identify areas where AI/NLP can be applied, and what are some of the applications?
Hoffmann said the reality is that the vast majority of firms are still very much in the discovery and diagnosis phase of applying AI/NLP technology in their operations. In this context, it is often viewed as a solution looking for problem. The solution, however, needs to be seen not just in terms of where AI can be applied, but importantly, where it can be applied to generate material value.
On identifying potential NLP applications – and the value they can generate – Hoffmann said there are two key questions to ask:
When considering a specific human worker, what are some repetitive, time-consuming workflows often involving the processing of high amounts of data/information that can be automated using NLP techniques? This approach is primarily a cost-saving play.
When considering a large repository of unstructured text documents, what are some specific data points contained within that if aggregated and extracted at-scale using NLP, could be used to generate high-value insight? This approach is a value-aggregating play.
On potential use cases for NLP, Hoffmann described three broad persona classifications:
The Reader (value-aggregating play): Document search and retrieval for research (e.g. due diligence). An example, in the context of SVB’s collapse, is searching huge volumes of text in publicly available documents (filings, earnings releases etc.) to identify relationships/risks between small cap banks and interest rates.
The Statistician (value-aggregating play): Market signal for investment decisions (e.g. portfolio balancing). Instead of processing a large amount of text to find a small amount of relevant information, this process involves quantifying a large amount of text and analyzing it to see what kind of signal emerges. An example is running analysis of a specific topic, such as force majeure, across the filings of the portfolio companies to identify why the topic has emerged and the level of exposure portfolio companies have.
The Bookkeeper (cost-saving play): Document processing for financial transactions (e.g. target company acquisition). An example is running an automated search of credit agreement documents, looking for specific values such as maturity dates and interest rates.
What does sentiment analysis look like using NLP?
S&P Global’s Patel described how the company is applying NLP through textual data analytics, which provides a way to look at earnings call transcripts and derive structured meaningful data from them.
There is a large amount of information from earnings calls that can be analyzed, including: the degree to which positive or negative news was revealed; how specific management was on topics; management’s choice of words; and how the attitudes of the analysts on the call differ from management.
From this structured data – at the overall core level as well at sub-levels, where the data is parsed into specific segments such as individual executives’ remarks or remarks made during the Q&A session – S&P Global creates sentiment scores, such as:
Net positivity score, which look at how many positive words were used versus negative words during a call.
Numeric transparency score, which looks at the ratio of numbers to words used, where a higher incidence of numbers is seen as more transparent.
Language complexity score, which analyses the language used and whether the speaker was using complex or simple language, which is typically preferred, as it likely means the presenter is not hiding behind jargon.
Patel shared four examples of sentiment scores:
US discount retailer, Dollar Tree: Net positivity score was significantly above industry average during Covid lockdowns – stores were kept open – and throughout last year as inflation soared and recession threatened.
Multinational luxury fashion brand holding company, Capri Holdings (owner of Versace, Jimmy Choo, Michael Kors): Net positivity score dropped significantly below the consumer industry average over the past six months due to rising inflation and consumer concerns over recession.
US materials company, Ball Corporation: The company’s managerial sentiment score dropped sharply from the CFO’s prepared speech – where it was on a par with industry peers – to the Q&A session, indicating a wide divergence in what was said in both scenarios. Ball’s credit default swap spreads started to widen in the weeks after the earnings call, highlighting the increased credit risk profile of the company.
Overall sentiment score of S&P 500 companies: Over 60 quarters, highly negative sentiment scores during the 2008/2009 global financial crisis and global pandemic. But sentiment post the pandemic bounced back more emphatically and rapidly than the GFC.
What’s Voya Investment Management’s story here? In what areas of the business is NLP being deployed?
Voya’s Andraos said Voya Machine Intelligence (VMI) has two roles.
The first is running eight fully systematic AI-based equity strategies – ranging from ESG momentum to small cap, international, large cap, etc. – which is achieved by “managing a zoo” of virtual analysts, traders, and portfolio managers. They fulfil the same job as their human counterparts and are equipped with high quality data and algorithms so they can do as well if not better at picking stocks than their human counterparts. The platform is extremely scalable, and customizable, enabling Voya to add products. It is also low-cost and capital light, combing the best of humans and machines.
I remember being a junior investment banker back in the 1990s. I know that ChatGPT style technologies can do a lot of the work I used to do back then. That's not a bad thing
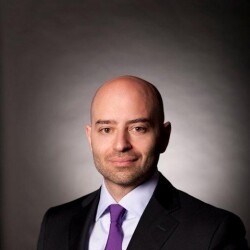
The second role is developing a platform to leverage AI for human teams. For human portfolio managers, whether they are on the fundamental side or on the quantitative side, VMI is operating in the middle, bridging all the different operations and destroying silos. The platform is completely transparent and was built from the ground up with fundamentals and quant concepts at its heart.
Key building blocks of Voya’s systematic investment process:
VMI uses many data sources composed of market and macro data, analyst estimates, company ownership, financials, ESG, etc. As a result, VMI has access to in-excess of 10,000 data points per company at any one point in time.
The next step is to generate features, which involves reducing the dimensionality of the data by distilling it into useful building blocks.
From there, VMI built its virtual analysts, traders, and portfolio managers. They are trained via neuro-symbolic AI, so called because the training process combines Bayesian networks with more traditional supervised machine learning techniques. The idea is to provide these virtual employees with 20 years of data allowing them to learn the patterns that separate good and bad investments.
Andraos gave two examples of where natural language understanding – a subset of NLP – is being deployed at Voya:
Stock attribution: At the end of every quarter, portfolio managers need to report to investors and provide info on which positions did well, and which didn't do well, and why. While these snippets are quite short, it takes time, and especially if the portfolio manager must do that for the five top and bottom positions across 10 products. VMI has completely automated this process by linking GPT-style models to its proprietary databases. To avoid the limitations of large language models (LLM) it’s key to separate the language layer from the memory layer and ground the LLM in factual content from curated sources.
ESG analysis: Voya has a database of ESG case studies covering cases such as the controversy around Amazon’s working conditions in 2021, Nikola Motor’s false advertising in 2020 or BP’s Deep Water Horizon disaster in 2010. While the database contains specific facts such as dates, duration of crisis, losses endured, etc... it lacks any context about what happened, how management reacted and the regulatory response, if any. VMI has developed a system that automates the research around each one of these cases by grounding an LLM around automated Google search results. The outcome is fully detailed research on each case with footnotes and references that can be clicked for further validation.
For EQT, how has it approached the use of NLP internally – at the general partner level – and more broadly, at the portfolio company level?
Casella said EQT started using AI and machine learning in general about five years ago. At the foundational layer, this involved organizing all the data available to the firm so that they could classify companies into their own taxonomy of sectors.
From there, EQT started ranking companies by training models to sort companies in order of relevance for the firm’s strategy. Part of this involved equipping deal professionals with key company data points, and providing a similarity search capability for aggregating information. Signal and forecasting tools were also developed.
As of last year, what with the commoditization of AI and the emergence of new skills from the latest language models, EQT has expanded the number and variety of use case applications.
Casella gave multiple examples of these:
Semantic search – Instead of interacting with data through filters, semantic search enables the user to ask questions and use natural language do that research. For instance, in M&A search, where you may have up to 50,000 companies of interest, the semantic search enables the user to ask questions that generate a refined universe of companies with qualities specific to the questions being asked.
Enhanced extraction power – Extraction involves asking specific questions of a large, unstructured set of information. For example, in a people database, questions can be asked about who, from a company or from a list of people, has a certain level of management experience in a specific sector. Today’s language models help filter this information better and more quickly.
Information generation – Equipping the firm’s deal team with the capability to automatically summarize information, translate and clean data, which enables them to process much larger sets of information more quickly.
Reasoning and ideation – Using a capability like ChatGPT to test a deal hypothesis by asking, for example, what five acquisitions company ‘A’ could make to expand its operations. The options are then fed back into the semantic search to identify possible new targets that the deal team may not have already considered.
Intelligent enterprise – Using new technology to make the entire enterprise more efficient and intelligent. For example, having developers deploy Google, GitHub co-pilot into their day-to-day tools. This frees and speeds up the developers, which means they can spend less time fixing bugs and more time working with deal professionals.
Banks have been some of the earliest movers on NLP. What has Bank of America been engaged in in this area, and how transformative is it proving?
Oddy said that NLP, AI, machine learning technologies are key tools in helping the bank increase its internal operating efficiencies and enhance the service it provides customers and clients across the retail and corporate and investment bank.
This involves actively leveraging the algorithms and using them to help interpret a vast range of complex unstructured messages that come in from its customers and clients for example via voice, text, chatbot, or the automated banking centre. Those messages are often then enriched with additional data.
That is something you can't really do today because it involves a sequence of different intents. But we want to enable those type of interactions on our platforms, and really take that natural language understanding to the next level
Some examples of where AI has been applied, include:
Retail banking – The bank has implemented an AI voice assistant, called Erica, that's available to all retail customers. That deployment has essentially created an intelligent, automated financial concierge service that’s open to customers 24/7.
Transaction banking – The bank has started to deploy similar AI technology in some of its banking products, such as intelligent receivables, where it can match outstanding invoices to the payments that are coming in.
In the future, the next move will be to shift away from individual elements of interaction, such as the direct request response typically through a virtual assistant, to engaging and acting on behalf of clients – whether a retail customer, corporate treasurer, or investment manager – in a more sophisticated way. For instance, instructing a virtual assistant to sell half of the number of shares held in a company before transferring the proceeds into an investment or savings account.
“That is something you can't really do today because it involves a sequence of different intents. But we want to enable those type of interactions on our platforms, and really take that natural language understanding to the next level,” said Oddy.